Money growing on trees - Easily overlooked cost-saving opportunities
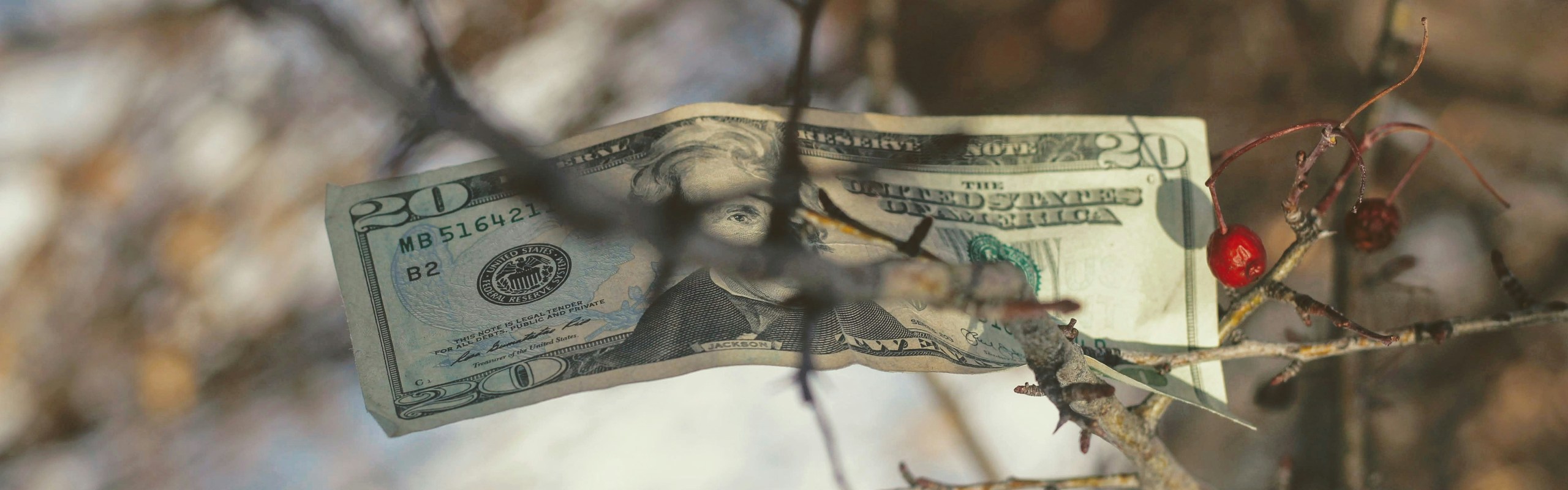
- Over-provisioning of resources
- Unshared cloud resources
- Excessive metrics cardinality
- Massive logs ingest
- Maintenance cost and overhead
- Resource-hungry tech stack
- Unrestricted scalability
- Barely used applications
- Summary
Distributed systems, and especially microservices are often a go-to solution for organizations seeking modernity, scalability and growth opportunities. What is often overlooked, though, is that as the scale of our infrastructure keeps growing, so does the scale of any inefficiencies introduced along the way. While few would find it worth investigating whether service’s upkeep could be cut from USD 200 down to USD 100 per month, the sum becomes non-trivial if we could cut the costs of running hundreds of microservices, several instances each.
Building distributed systems, including cloud-based microservices, is a challenging and rather expensive journey - even if done efficiently. If we overlook some of the major inefficiencies, however, the high cost may become simply prohibitive, necessitating a mindful approach. In this post, we will explore a number of examples where teams or organizations realized (or not) they are over-spending on their infrastructure at scale:
- Over-provisioning of resources,
- Unshared cloud resources,
- Excessive metrics cardinality,
- Massive logs ingest,
- Maintenance cost and overhead,
- Resource-hungry tech stack,
- Unrestricted scalability,
- Barely used applications.
Over-provisioning of resources
It is deceptively easy for Software Engineers to provision compute resources in the cloud. In fact, this is the very reason why I personally consider cloud computing superior to classic ways of managing infrastructure - more on this in Cloud Transformation misconceptions. In case of cloud infrastructure from third-party vendors, it is in their best interest to make provisioning of resources even easier, with dedicated dashboards, plentiful integrations, a wide range of compute resource types, usage-based billing and whatnot.
As helpful as it is, it also makes over-provisioning of resources exceptionally easy - after all, if something is so easy to do, few would think how much it actually costs to do so. Individually, such over-provisioning might be negligible and not worth investigation, however at scale it often incurs excessive costs which cannot be overlooked.
Unshared cloud resources
The problem here is primarily that since each individual application had its own, dedicated compute resources, they were all deployed with prepare for the worst mindset. Applications might be provisioned with plenty of CPU power simply because they are demanding at startup time. During runtime, though, they could barely use any of their assigned resources. As they would not share resources with each other, this would be done over and over again.
There are two primary ways to avoid this conundrum:
- Orchestrate your containers on shared compute infrastructure - with Kubernetes, Nomad or similar,
- Attempt to replace expensive, overpowered infrastructure with more cost-efficient alternatives, at the expense of lower stability and/or longer startup times.
Excessive metrics cardinality
Our investigation with the SRE team has shown that due to generous labelling, the cardinality of our metrics reached hundreds of thousands, if not millions already. When we started investigating actual metrics-based dashboards and queries we actually used for monitoring, it turned out the cardinality we truly needed was a fraction of this - maybe several thousand.
What this meant was that without cutting on cardinality of ingested data, we would be spending considerable sums on series which we would never use directly - only aggregated with other, similar series. This also meant additional, needless processing, possibly impacting performance. Filtering out unused label dimensions in metrics collectors helped us cut down on the numbers significantly.
Massive logs ingest
There are several reasons why excessive logging:
- From the costs perspective, it leads to over-spending on log processing provided by 3rd party vendor, or forces the organization to maintain more powerful log processing solution with more hardware resources, which also costs money.
- On the other hand, applications churning out plenty of not-so-useful logs essentially generate noise that makes troubleshooting harder. Spending time on writing queries to filter out redundant logs - rather than irrelevant for a particular search - is a good indicator our logs might be of poor quality.
- Lastly, the sheer volume of logs may lead to running out of processing quotas with our vendor, or to running out of storage space, forcing us to cut down on logs retention. The volume also contributes to more time-consuming and costly logs processing. Worst case scenario is when after running out of quotas, the log server would simply drop new logs altogether, regardless of their criticality.
All in all, it is better to emit fewer logs providing more condensed, and well-formatted (for ease of processing) logs that to try to log everything, or log pieces of information we will not only find useless, but we will actively avoid as they prevent us from gathering useful insights.
Maintenance cost and overhead
In some aspects, microservices make it way easier to maintain extensive software - smaller applications are arguably easier to run, configure and work on if considered in isolation from other parts of the system. On the other hand, however, they can drastically increase the maintenance cost, and maintaining dependencies of each individual microservice often gets neglected. Another contributing factor is lack of proper tooling - without Dependabot or other automated patching tools, it becomes especially painful to upgrade vulnerable dependencies across dozens, if not hundreds of applications.
Resource-hungry tech stack
While Java by itself performs quite well, especially with all the optimizations that had been added to JRE over the years, it happens to be quite resource hungry when paired with massive, runtime-intensive frameworks such as Spring / Spring Boot. Once the application is fully started, it does not require much in terms of computing power, meaning that with shared vCPU resources it is possible to optimize CPU resource utilization. On the other hand, high usage of RAM is rather consistent, and makes it difficult to avoid over-provisioning of compute resources for the sake of fitting all the Java applications in.
You can read more on Java inefficiencies in cloud computing in Does Java make a good fit for microservices? - this is not to say, however, that Java is the only programming language. It is more related to some of it’s Virtual Machine defaults, and resource-hungry frameworks such as Spring / Spring Boot. Simply avoiding a particular programming does not guarantee applications would magically become efficient.
Unrestricted scalability
Scalability is an immensely powerful feature of cloud-based microservices, as it allows organizations to adapt to changing demand for their services. It does not mean, however, that this scaling should be completely unrestricted - sometimes it simply makes sense to put a cap on how much an infrastructure or software components can scale up or out. Imposing some limits on resource utilization by the clients or Software Engineers can save us headache when invoices are sent.
In some cases, scalability is not even strictly needed. One example of such needless scaling could be situations where a microservice starts to choke, but the real problem is its underlying database’s throughput rather than the application itself. In such cases, scaling out an application could even be counter-productive, as it could put even more strain on an already struggling database. It could also happen that the service’s API is being abused by another application or some third party.
More practical solution to such issues would be to carry out an audit and act accordingly to the root cause:
- Introduce caching (if possible) to reduce needless processing,
- Impose rate limits to reduce the risk and scale of abuse,
- Address bottlenecks case-by-case:
- If an application runs out of DB connection pool, increase the pool’s size to allow serving more simultaneous requests by the same instance of application,
- If an underlying database struggles, investigate its load and whether they way it is used could be optimized - for instance, by improving indexes,
- If it is not feasible to further optimize a single database instance, consider replication to offload read-only transactions, or a database cluster for overall scalability improvement,
- Ultimately, when the application’s capacity truly becomes a bottleneck, it is time to scale it out.
Barely used applications
The problem is that with dedicated resources reserved for a particular application, if they are not used by this particular application, nobody else can come and put them to a better use. Likewise, since they were already provisioned by the cloud vendor, they would be billed regardless of the extent to which they were truly utilized.
Deploying minuscule, low-traffic applications with dedicated, powerful resources is a highly inefficient approach, and if it becomes widespread it can incur non-trivial costs for compute resources which cannot ever be fully utilized.
Summary
While by no means exhaustive, these examples of cost inefficiencies should give an idea of how cost inefficiencies manifests themselves in distributed systems, most notably in cloud-based microservice architecture, and how they could be addressed.
Moreover, these particular case studies show that cost reduction does not necessarily mean giving up the quality - on the contrary, in case of distributed systems it is not uncommon for cost optimization and quality improvements to go hand in hand. If the root cause of cost inefficiency is over-engineering, over-provisioning or unsuitable architecture, addressing these issues can be in fact beneficial for Software Engineers building and maintaining these systems.